Please take a look at our new preprint “The combination of Hebbian and predictive plasticity learns invariant object representations in deep sensory networks.”
https://biorxiv.org/cgi/content/short/2022.03.17.484712v2
In this work led by Manu Srinath Halvagal we argue that Hebbian plasticity could be the essential ingredient that allows the brain to perform self-supervised learning without the problem of representational collapse. Feedback is welcome, as always!
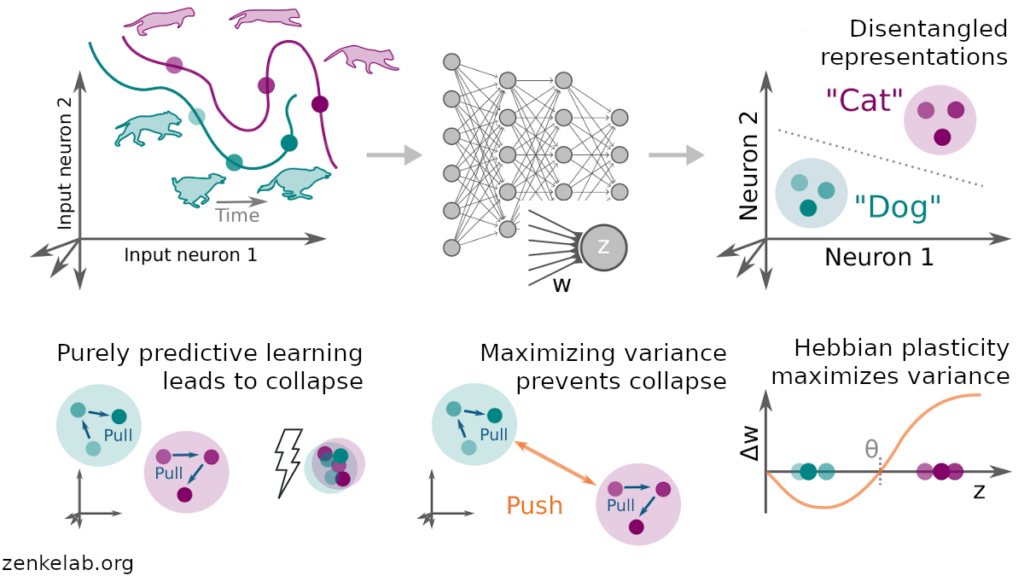
Our work conceptually links negative-sample-free self-supervised learning that rely on neuronal decorrelation (Barlow Twins) and variance regularization (VICreg) to a Hebbian plasticity model, which shares several notable similarities with, but also generalizes, BCM theory.
We show that in a layer-local learning setting (greedy learning), the plasticity model disentangles object representations in deep neural networks.
Finally, the same model faithfully captures neuronal selectivity changes of in-vivo unsupervised learning experiments in monkey IT (Li and DiCarlo, 2008).