Gitta Kutyniok, the Bavarian AI Chair for Mathematical Foundations of Artificial Intelligence and her team at the LMU organized an inspiring workshop on spiking neural networks with a fantastic lineup of speakers including Wolfgang Maass, Sander Bohte, Wulfram Gerstner, Dan Goodman, Emre Neftci, and many others. It was a great opportunity to showcase the most recent work from our group on the topic:
- Spiking Datasets: Cramer, B., Stradmann, Y., Schemmel, J., & Zenke, F. (2022). The Heidelberg Spiking Data Sets for the Systematic Evaluation of Spiking Neural Networks. IEEE Transactions on Neural Networks and Learning Systems, 33(7), 2744–2757. https://doi.org/10.1109/TNNLS.2020.3044364
- Theory of SNN learning algorithms: Gygax, J., & Zenke, F. (2025). Elucidating the Theoretical Underpinnings of Surrogate Gradient Learning in Spiking Neural Networks. Neural Computation, 1–40. https://doi.org/10.1162/neco_a_01752
- SNN initialization: Rossbroich, J., Gygax, J., & Zenke, F. (2022). Fluctuation-driven initialization for spiking neural network training. Neuromorphic Computing and Engineering, 2(4), 044016. https://doi.org/10.1088/2634-4386/ac97bb
- SNN training on neuromorphic hardware: Cramer, B., Billaudelle, S., Kanya, S., Leibfried, A., Grübl, A., Karasenko, V., Pehle, C., Schreiber, K., Stradmann, Y., Weis, J., Schemmel, J., & Zenke, F. (2022). Surrogate gradients for analog neuromorphic computing. Proceedings of the National Academy of Sciences, 119(4). https://doi.org/10.1073/pnas.2109194119
- SNNs for BMI applications: Liu, T., Gygax, J., Rossbroich, J., Chua, Y., Zhang, S., & Zenke, F. (2024). Decoding Finger Velocity from Cortical Spike Trains with Recurrent Spiking Neural Networks. 2024 IEEE Biomedical Circuits and Systems Conference (BioCAS), 1–5. https://doi.org/10.1109/BioCAS61083.2024.10798222
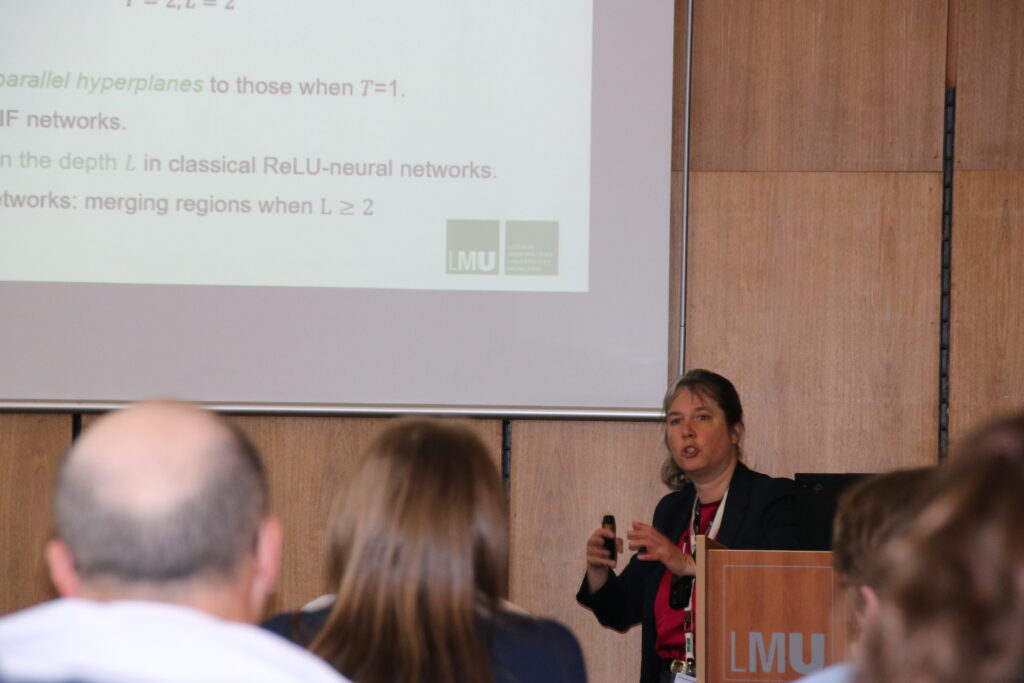
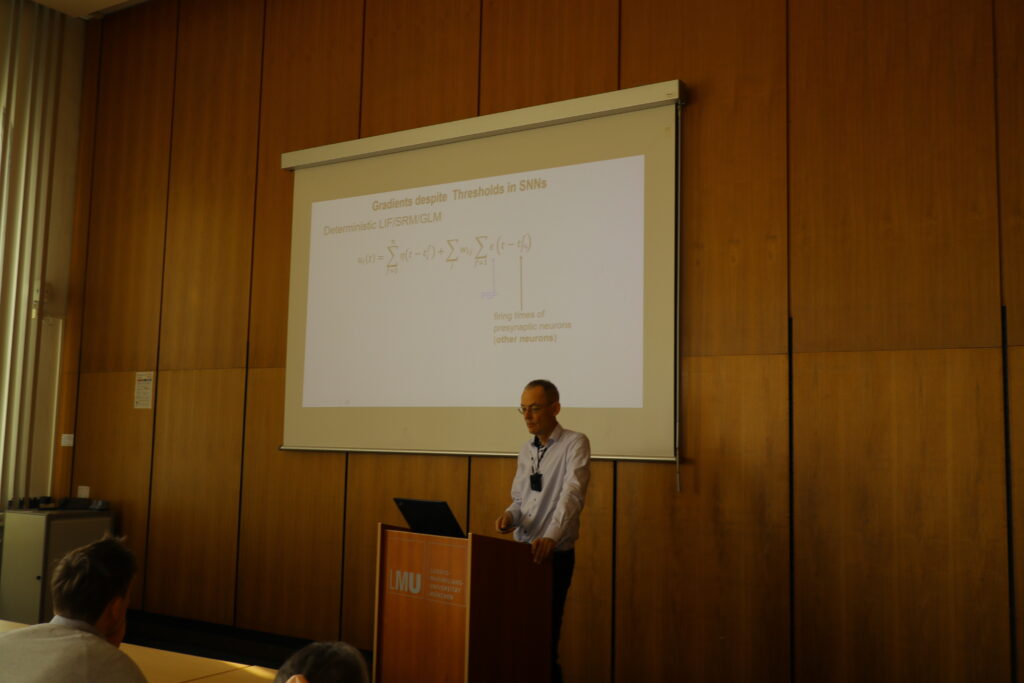
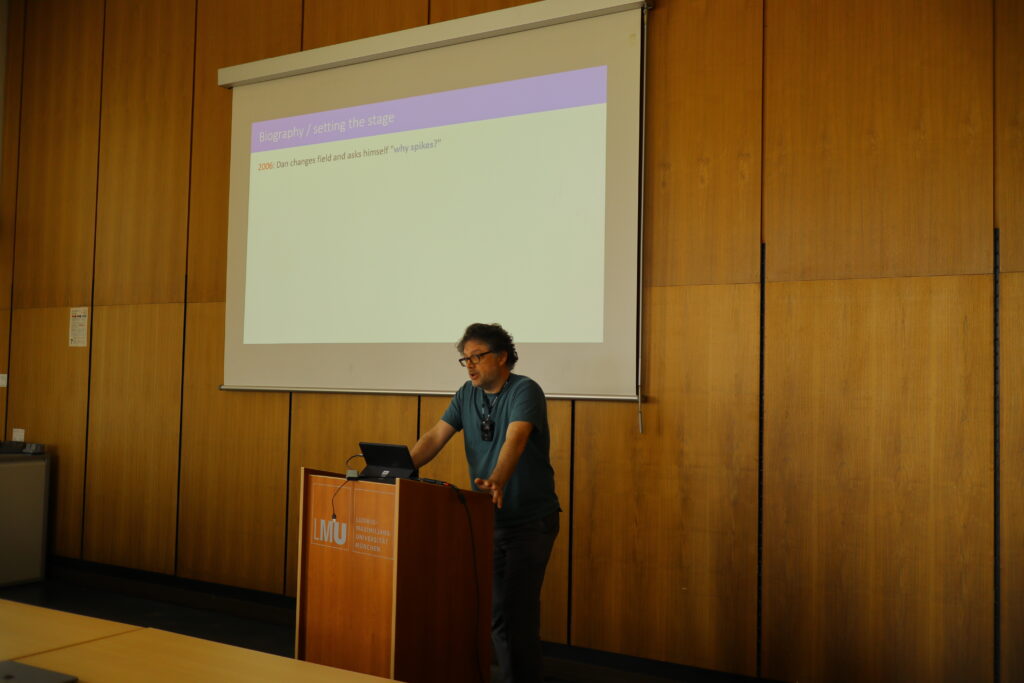
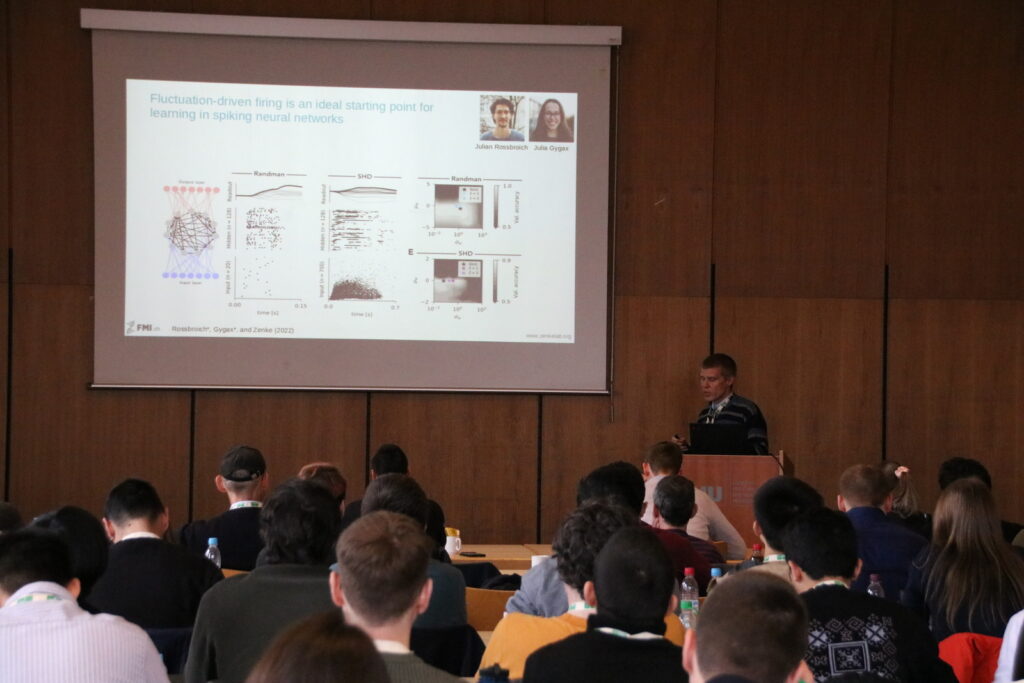
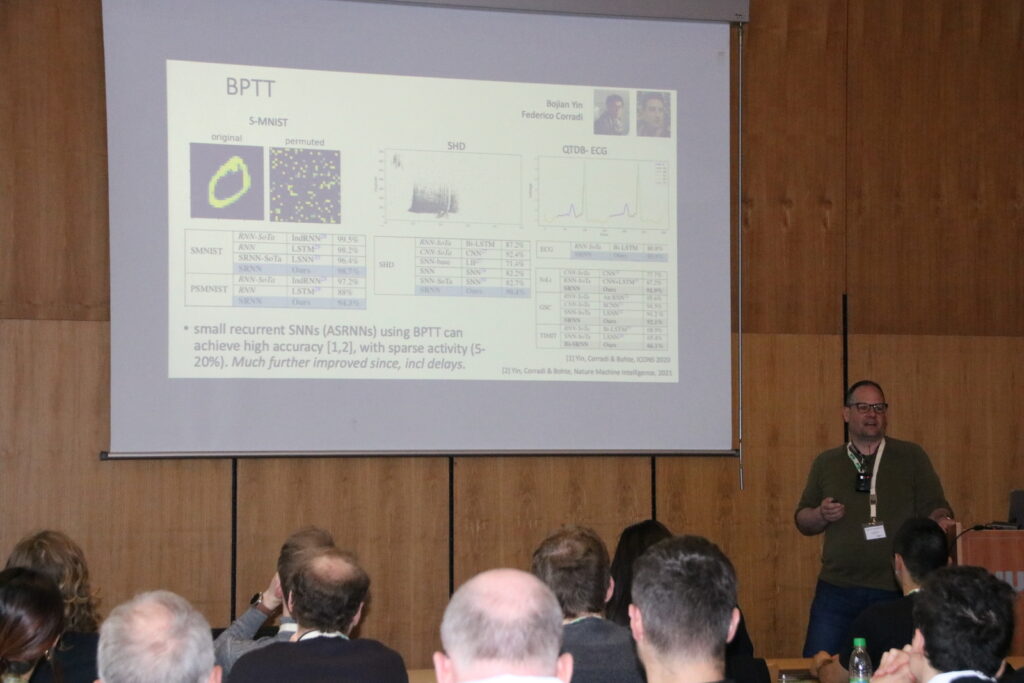
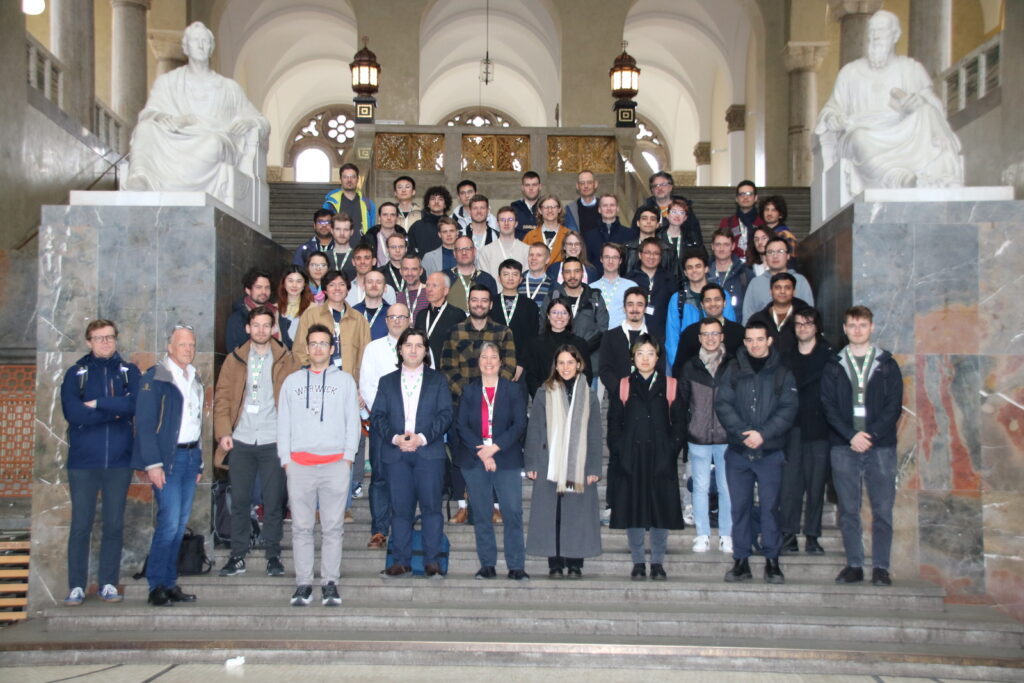