Why does the brain maintain such precise excitatory-inhibitory balance?
Our new preprint led by Julian Rossbroich explores a provocative idea: Small, targeted deviations from this balance may serve a purpose: to encode local error signals for learning.
https://www.biorxiv.org/content/10.1101/2025.05.12.653626v1
Existing theories of bio-plausible learning and credit assignment often rely on segregated dendrites to encode neuronal errors. However, not all neurons have morphologically well-separated dendrites while data-driven plasticity models seem at odds with such error modulated learning.
We propose feedback signals may target interneurons to transiently disrupt E/I balance. These controlled deviations can efficiently encode local error signals, allowing to guide plasticity without the need for special dendritic morphology.
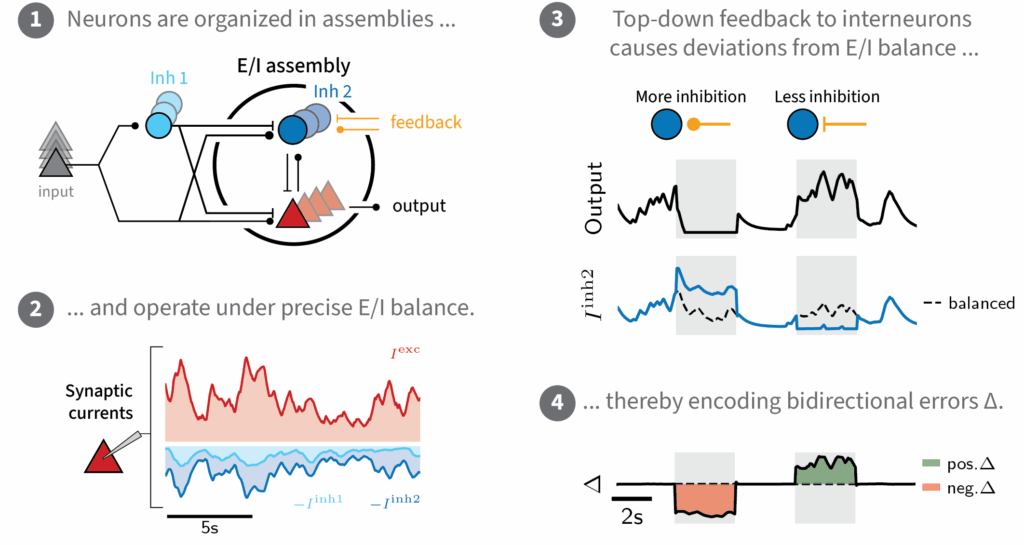
We formalize this idea as Balance-Controlled Plasticity (BCP), a plasticity framework grounded in adaptive control theory, which simplifies to a Hebbian-like learning rule modulated by recurrent inhibition. This learning rule is both biologically plausible yet powerful.
In multi-layer networks, BCP enables online learning without separate training phases and segregated dendrites, while only using feedback-driven E/I balance perturbations.
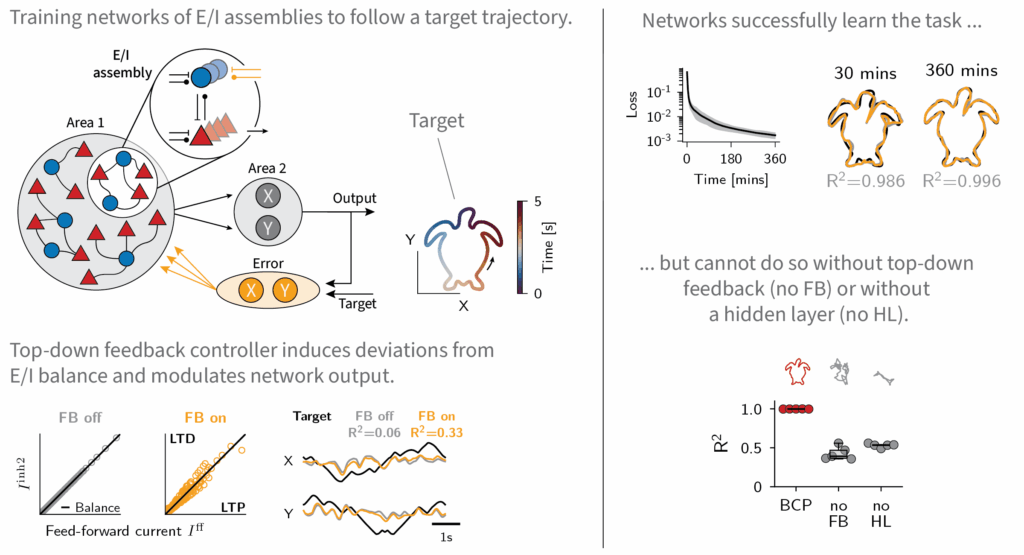
BCP does not only work. Trained networks also replicate in-vivo dynamics of SOM and VIP interneurons during motor learning and fear conditioning experiments. Our model takes a step toward linking neuronal circuits with plasticity and behavior.
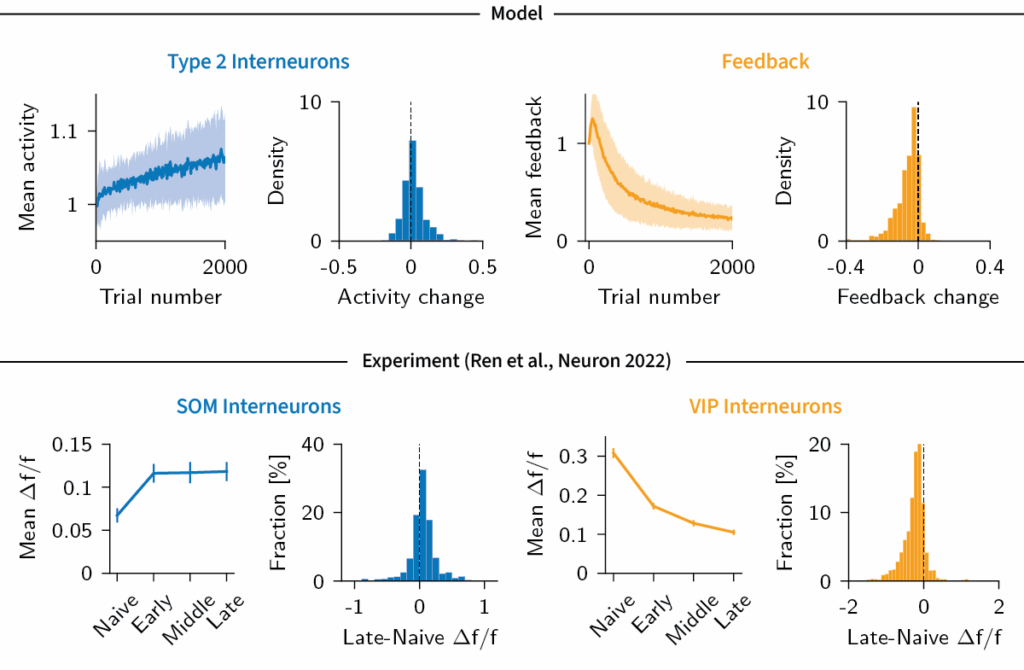