In Julian’s new paper “Dis-inhibitory neuronal circuits can control the sign of synaptic plasticity“ accepted at NeurIPS we look at how to reconcile normative theories of gradient-based learning in the brain with phenomenological models of Hebbian plasticity.
Preprint: https://arxiv.org/abs/2310.19614
Code: https://github.com/fmi-basel/disinhibitory-control
Models of bio-plausible credit assignment rely on neuronal error signals to change the sign of plasticity. Experiments suggest the sign depends on postsynaptic firing rate, voltage, or calcium. How does this fit? We show that inhibitory microcircuits could resolve this conundrum.
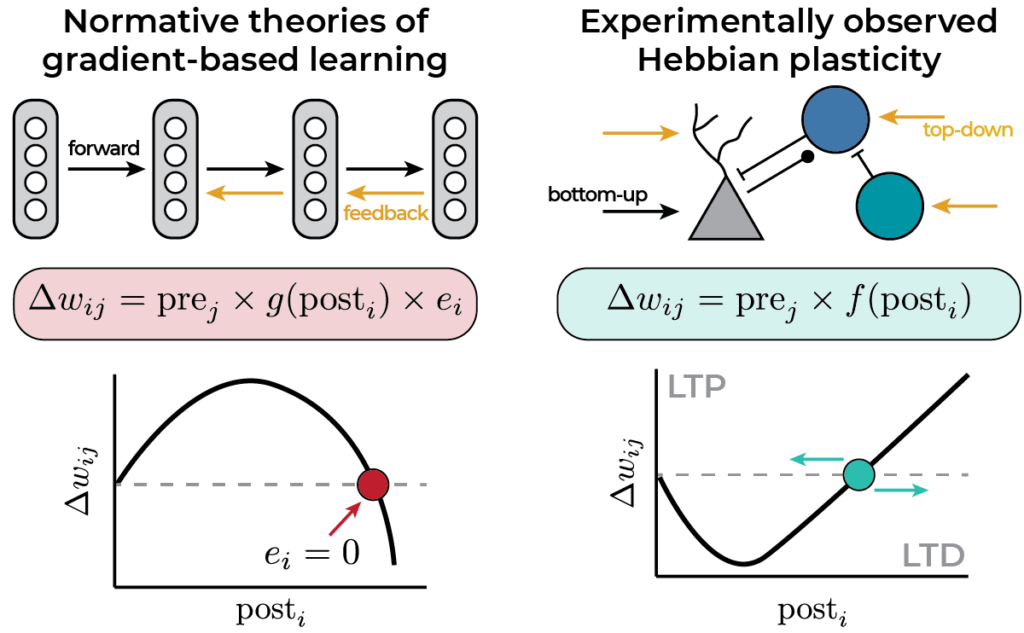
We extend work on Deep Feedback Control (Meulemans et al. 2021) with dis-inhibitory neuronal circuits and demonstrate that they can control the sign of plasticity, closely in line with experimental results on dis-inhibitory gating of learning (Krabbe et al. 2019; Williams and Holtmaat 2019).
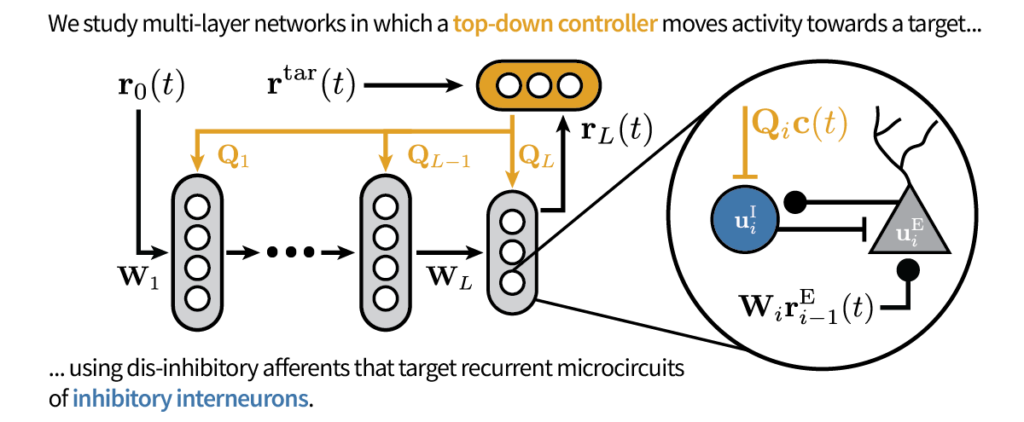
In our model, the error is encoded in the recurrent inhibition. We derive a Hebbian learning rule that decodes the error from inhibitory currents using a dual threshold mechanism: one reminiscent of the BCM rule and one inhibitory current-dependent.
The resulting learning rule does error-driven learning for suitable top-down feedback. Interestingly, the inhibitory threshold stabilizes Hebbian plasticity even in the absence of top-down feedback.
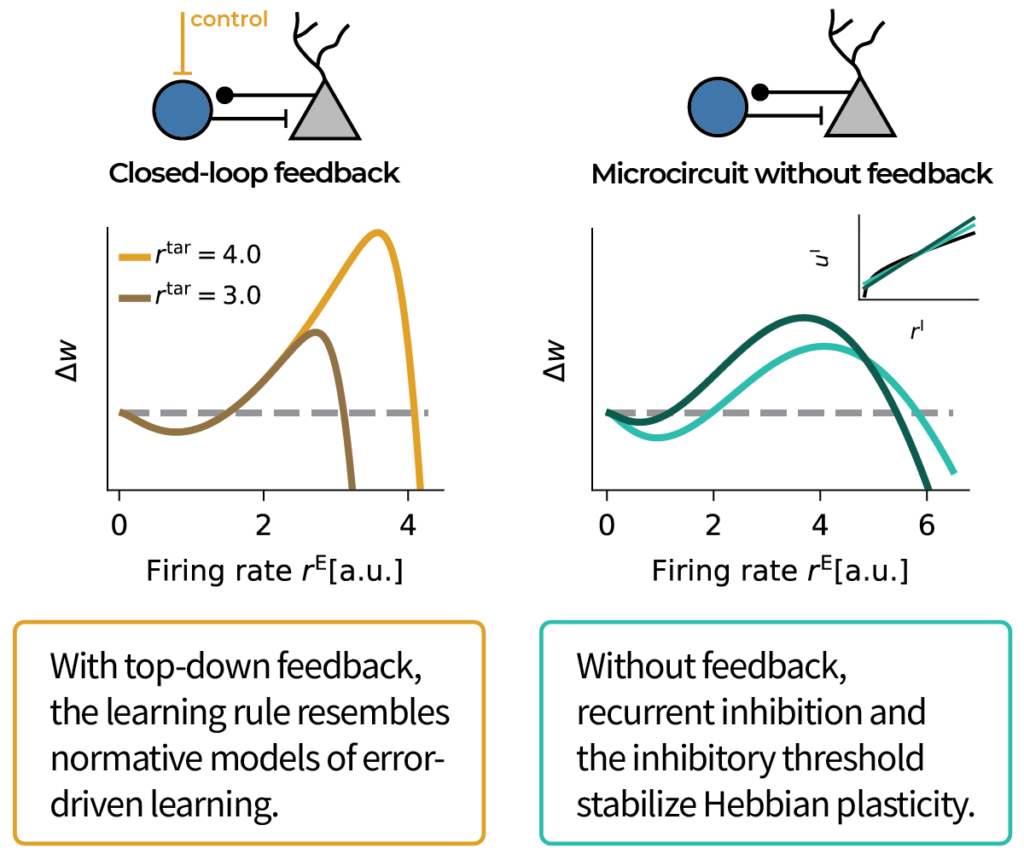
In contrast, when we disrupt recurrent inhibition to simulate in vitro slice conditions, the learning rule resembles experiments. Moreover, our model predicts that injecting inhibitory current while keeping the firing rate constant should bias plasticity towards LTD.
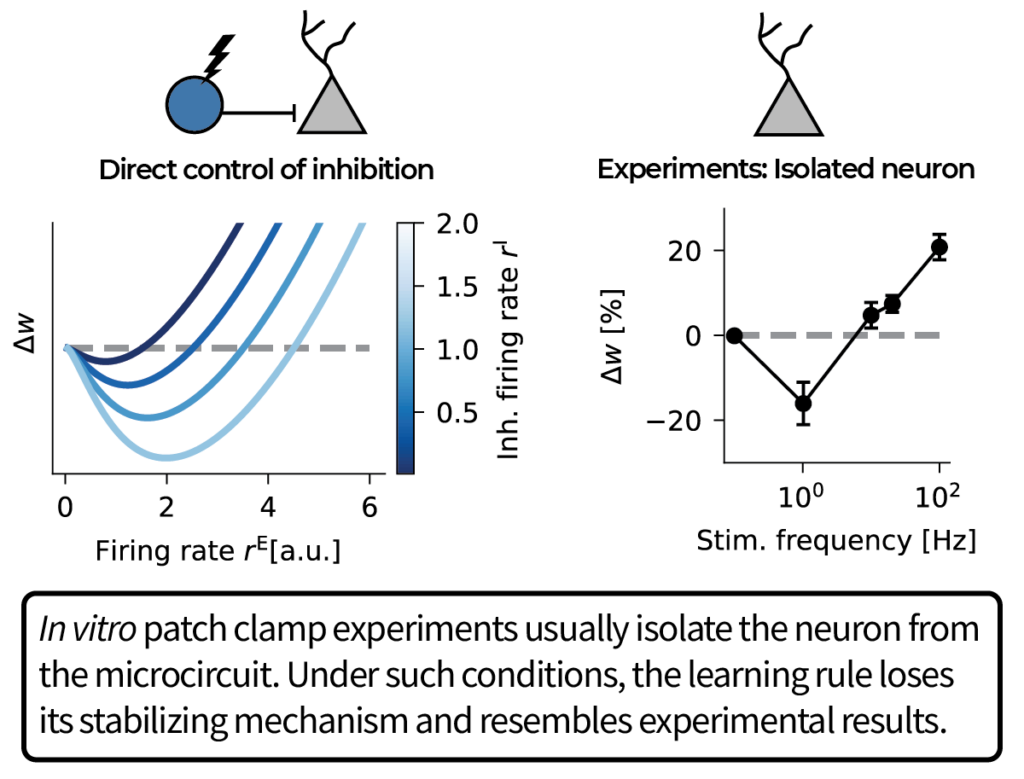
Finally, we show that dis-inhibitory control can effectively perform credit-assignment in multi-layer networks with performance close to backprop.

Thus, we propose that inhibitory circuits could encode neuron-specific error signals and control the sign of plasticity with an inhibition-dependent Hebbian rule. Our model puts forward a possible explanation why error-driven plasticity has been elusive in experiments.