We just put up a new preprint https://www.biorxiv.org/content/10.1101/2020.06.29.176925v1 in which we take a careful look at what makes surrogate gradients work.
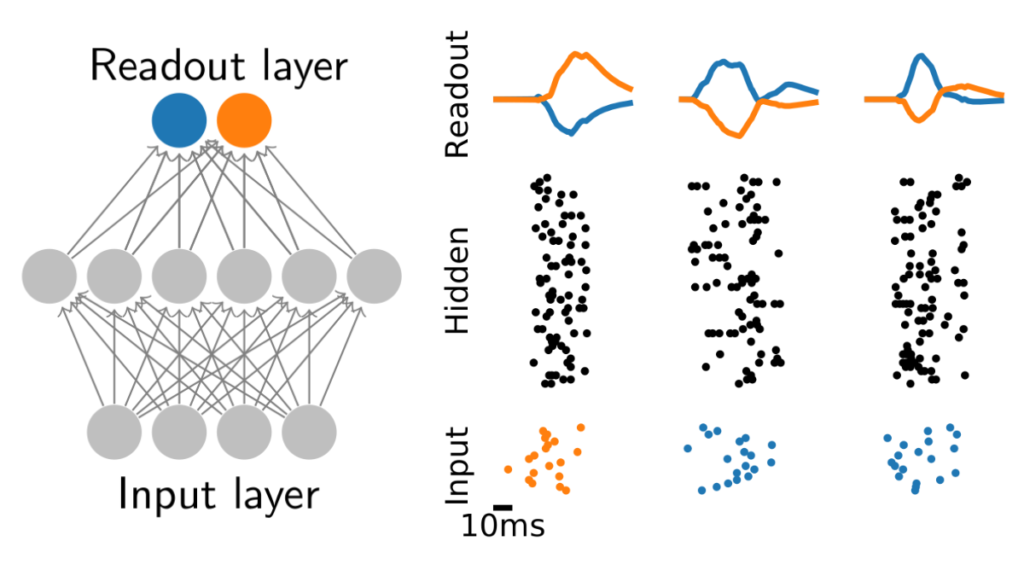
Spiking neural networks are notoriously hard to train using gradient-based methods due to their binary spiking nonlinearity. To deal with this issue, we often approximate the spiking nonlinearity with a smooth function to compute surrogate gradients. But due to freedom of choice in the approximation, surrogate gradients are not unique. Hence, we wondered whether there are choices for the surrogate function that are better than others. To investigate this question, we systematically varied both its shape and scale.
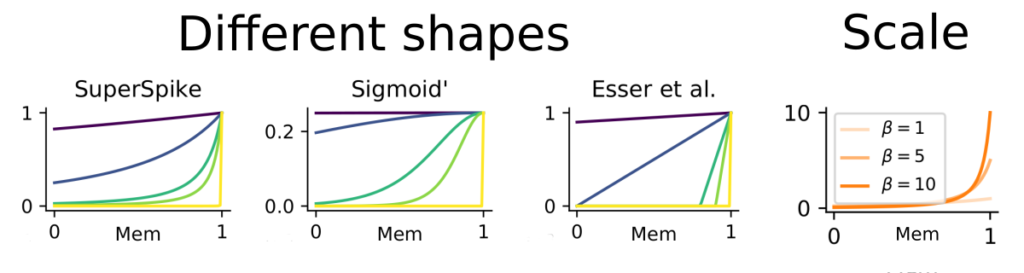
To probe the influence of different shapes and scales, we trained spiking neural networks on a range of different datasets ranging from synthetic data drawn from smooth random manifolds to real-world datasets. We found remarkable robustness of surrogate gradient learning in spiking neural networks concerning the shape of nonlinearity we used, but not to its scale.
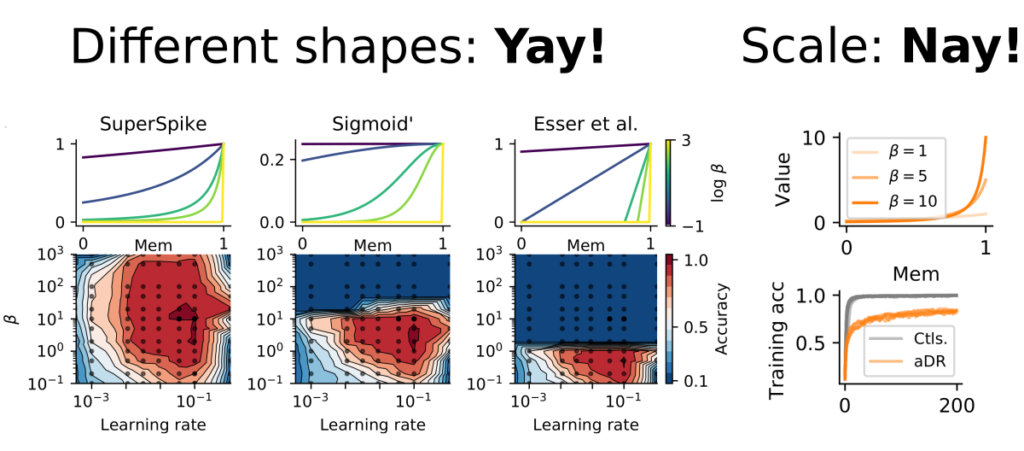
Finally and reassuringly, we show that these results are not affected by enforcing sparse spiking activity through regularization. Therefore, we think surrogate gradients offer an exciting new approach to studying the computational principles of spiking neural networks in-silico.
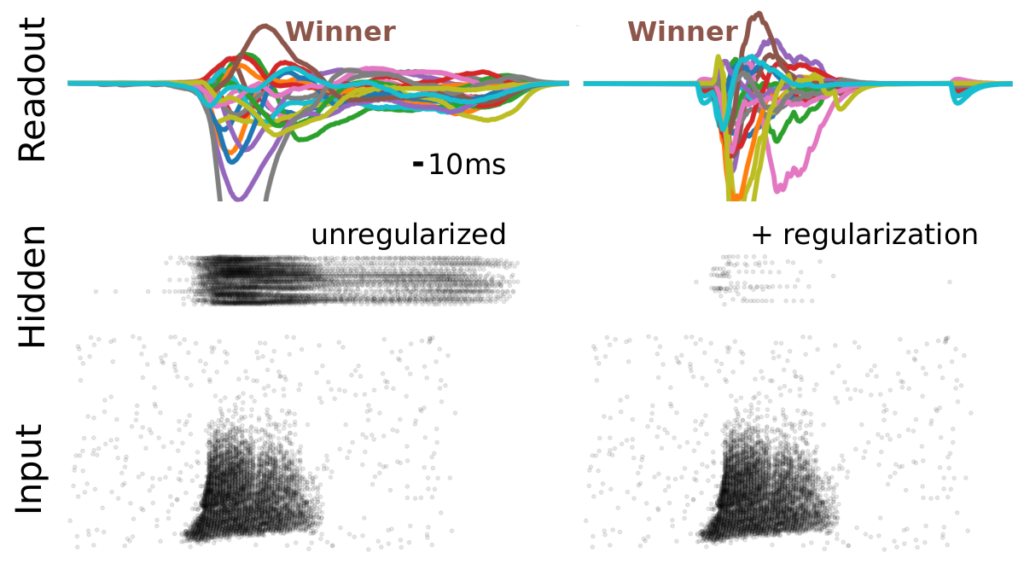